Note: First published in 2015, this article remains one of the most popular on our blog. We updated this post in July of 2020 to reflect the current volume of protein structure and sequence data. Spoiler alert: the availability of new protein sequence data continues to far outpace the availability of experimental protein structure data, only increasing the need for accurate protein modeling tools.
Why Structure Prediction Matters
Steve Darnell started his career as a structural biologist and computational biologist at the University of Wisconsin, developing computational models to predict binding hot spots in protein-protein interactions. In 2008 he joined DNASTAR and helped launch Protean 3D for protein sequence and structure analysis, and later, NovaFold for protein structure prediction. We recently sat down with Steve to learn more about the importance of protein modeling.
Why is it so important to understand a protein’s three-dimensional structure?
A protein’s biological function is dictated by the arrangement of the atoms in the three-dimensional structure. This could be the arrangement of catalytic residues in an active site or how a protein interacts with other proteins for structural or other regulatory purposes. Having a protein structure provides a greater level of understanding of how a protein works, which can allow us to create hypotheses about how to affect it, control it, or modify it. For example, knowing a protein’s structure could allow you to design site-directed mutations with the intent of changing function. Or you could predict molecules that bind to a protein.
What are some of the challenges of solving protein structures?
From an experimental point of view, the largest challenges are cost, time and expertise. Solving structures using crystallography and NMR requires extremely specialized training, a high degree of skill, and a lot of luck. The cost of solving a new, unique structure is on the order of $100,000.
Given the difficulty of solving an experimental structure, and considering the rate at which new protein sequences are discovered, it has become clear that with today’s technology, we will not solve structures for all the new proteins being identified and sequenced.
Comparing the number of protein sequences in UniProt to the number of known structures in the PDB (Figure 1), we see over 1700 times more sequences than structures. When this article was first published 5 years ago, that difference was only 400 times more. Since the number of new sequences continues to grow exponentially faster, that gap is here to stay and will only become wider over time.
Finding alternative ways to predict a protein structure becomes more and more important as this gap increases.
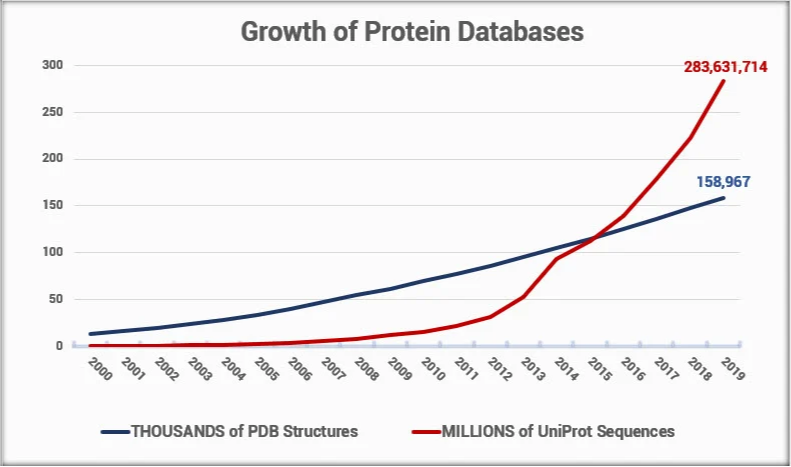
Figure 1. Growth of protein sequence and structure databases over time
How can protein structure prediction programs help bridge this gap?
Many tools for protein structure prediction rely on homology modeling. This works by using sequence alignment to identify proteins that have a high degree of sequence similarity in the Protein Data Bank. These methods work well for proteins with at least 70% sequence identity. But relying on sequence similarity alone has its weaknesses. As you get closer to 50% sequence identity, it becomes difficult to select templates. And as you get closer to the 30% sequence identity level, or the “twilight-zone,” it becomes exceedingly difficult, because any two random pairs of proteins can have this level of sequence identity.
The benefit of NovaFold is that it uses a hybrid approach that uses protein threading to select templates. Protein threading uses predicted secondary structure, predicted solvent accessibility, and predicted internal contacts, in addition to sequence similarity. This has the benefit of being able to find more distant relationships than sequence similarity alone, because we are ultimately looking for structural similarity. NovaFold goes a step further by using a collection of templates for modeling and using ab initio folding techniques to enhance the quality of the predicted structure.
How can protein structure prediction programs help bridge this gap?
In structure prediction, it is important to have tools to understand how much confidence you can have in predicted models. This is why NovaFold provides global and localized confidence scores for all models (Figure 2).
reasonable for doing protein design experiments, creating site-directed mutations, and predicting binding sites; and models with the highest confidence scores are appropriate for workflows like ligand and small molecule docking, virtual drug screening, and protein-protein docking.
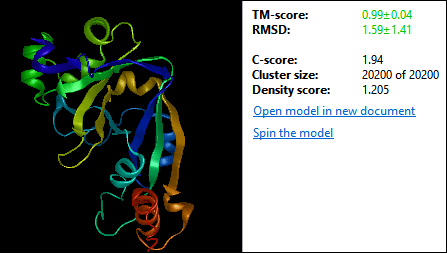
Figure 1. Growth of protein sequence and structure databases over time
In the end, people will always ask if experimental evidence is needed to prove the predicted models are correct. Experimental validation will still be important, but that doesn’t have to be experimental structure determination.
What are some of the current and future applications of protein structure prediction?
Predicted models are already being used for drug screening using homology modeling in well understood systems, such as kinases.
There is a lot of interest in structure prediction as a screening process for proteins that are not tenable for experimental determination. For example, companies working on antibody development can generate thousands of antibody sequences in response to targets. They need to screen out antibodies that don’t have desired characteristics as quickly as possible. One way to improve this quick-to-fail mentality is to use structure prediction to predict properties of a protein fold based on the amino acid sequence.
These tools will also be used for protein engineering. I think we’re going to reach a level of accuracy where you can predict a structure and use that to design increased or reduced affinity to binding partners without the need for an experimental structure.
People may also want to model genetic variation into a protein structure, from site-directed mutations to large insertions and deletions, to predict the structural, and by extension, the biological effects of those variants.
Having tools to probe the structure-function relationship for individual targets is going to become even more important as we try to reduce the time and cost required to do these studies. At DNASTAR, we’re working to make these tools accessible to everyone.
Predicted models are already being used for drug screening using homology modeling in well understood systems, such as kinases.
There is a lot of interest in structure prediction as a screening process for proteins that are not tenable for experimental determination. For example, companies working on antibody development can generate thousands of antibody sequences in response to targets. They need to screen out antibodies that don’t have desired characteristics as quickly as possible. One way to improve this quick-to-fail mentality is to use structure prediction to predict properties of a protein fold based on the amino acid sequence.
These tools will also be used for protein engineering. I think we’re going to reach a level of accuracy where you can predict a structure and use that to design increased or reduced affinity to binding partners without the need for an experimental structure.
People may also want to model genetic variation into a protein structure, from site-directed mutations to large insertions and deletions, to predict the structural, and by extension, the biological effects of those variants.
Having tools to probe the structure-function relationship for individual targets is going to become even more important as we try to reduce the time and cost required to do these studies. At DNASTAR, we’re working to make these tools accessible to everyone.
Want to learn more? Click the button below to visit our Protein Structure Prediction page. There, you can access videos and case studies about using NovaFold for protein modeling or schedule a demo with our in-house experts.
© 2025 — DNASTAR Privacy Policy